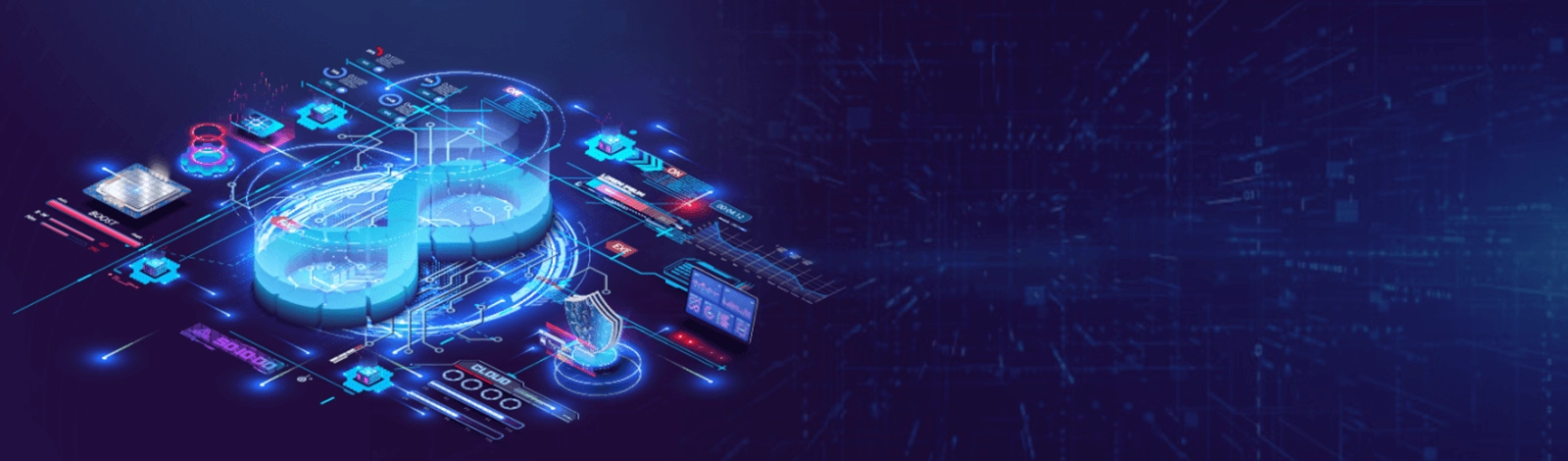
Marketers have commonly harnessed AI for enhancing customer experience and automating repetitive tasks. However, with the AI market projected to reach over 107.5 billion by 2028, the industry is unfolding exciting opportunities for marketers to stay a step ahead of their customers. Especially as recession looms on the horizon, businesses will be looking at slicing budgets, making it tables stakes to adopt accurate targeting techniques, the responsibility of which falls squarely on the shoulders of marketers.
The next frontier in marketing will be staying ahead of the customers by predicting their next move with AI. This article dives into how AI is changing the face of predictive analytics.
Predicting Customer’s Next Move With Predictive Analytics
Predictive analytics is a method that uses historical customer data, including geographical data, past customer habits, and purchase history, to drive future predictions.
While the concept and practice are not new to the marketing world, the advancement in AI is changing how marketers leverage predictive models, extract insights, and utilize the same to enhance marketing initiatives.
One of the most prominent benefits of AI is the ability to parse an enormous amount of data sets to filter the most relevant information and produce actionable insights, especially in today’s hyper-competitive world, where the proliferation of channels and data they produce can be overwhelming to deal with. Research shows that 50 % marketers use predictive analytics to forecast customer trends.
As AI is advancing, it can capture more nuanced sentiments. For example, earlier versions of AI focused on sentence fragments such as “good” or “bad” to determine customer emotions. However, they could not detect sarcasm where the mention of positive words still meant negative feedback. That’s where AI today is stepping up the game. It is not only able to pick up words but also understand the intent to identify the message behind it correctly.
Here are a few ways by which AI is taking predictive analytics to another level:
-
Creating personalized experiences
Based on customers’ purchase history, marketers can analyze their age, gender, demographic location, and other aspects, making their shopping journey personalized, and increasing sales. This can be further used to make future predictions.
One instance is where Netflix uses predictive analytics to understand its customer’s behaviors and past viewing patterns to suggest shows under the “Because You Watched…” section, which increases viewer engagement and attention rate on the platform.
Additionally, utilizing data derived from predictive analytics, and sentiment analysis allows marketers to better understand each buyer persona, their buying preferences, stay connected with their evolving requirements, and deliver a more personalized and meaningful omnichannel experience.
-
Reducing churn rate
Combining findings from sentiment analysis and predictive modeling, marketers can analyze customers’ feedback on the business’s current product and their sentiments around it. This can be used to identify a customer who is having an unfavorable experience and target them with specific messaging to prevent them from churning.
-
Generating lead scores
Piles of data can be transformed into lead scores that allow marketers to distinguish repeat and loyal customers from those on the verge of churning, and deliver personalized messages.
-
Making sentiment-based decisions
Using sentiment analysis, marketers can track changing customer behavior and trends. This allows businesses to customize their current offerings and introduce a new range of products to meet evolving customer requirements and ensure business continuity.
For instance, Skullcandy, a leading audio device brand,
uses predictive analytics and combined sentiments from across channels to enhance its new range of products and predict return rates on new products. -
Revolutionizing healthcare
Healthcare industry can utilize the data generated from the latest technology, such as wearables, to curate personalized and timely healthcare treatment plans. For instance, Fitbit, a leading wearables company encourages users to monitor and share their data so it could be used for better predicting and assisting users with their healthcare requirements.
-
Enhancing supply chain efficiencies
The challenge of overstocking and understocking can be prevented by forecasting demand by generating real-time updates using predictive analytics. Additionally, companies can leverage AI for predictive maintenance and ensure it doesn’t affect the customer experience. Finally, predictive analytics can be used to optimize inventory and reduce costs and enhance overall supply chain efficiency.
This is only the tip of the iceberg, the insights AI can collate, assess, and comprehend is a goldmine for marketers. Understanding the context of data is one of the most standout features of AI as it reduces the dependence on data analysts to comprehend data, who can then focus on more high-value work, such as creating predictive models that marketers can utilize.Here are some of the models that can be created utilizing predictive modeling:
- Neural network: A neural network mimics the human brain by creating a model that connects the dots between data sets to make sense of it. One of the most prominent uses of neural networks is customer segmentation. Neural networks work well by grouping customers based on their characteristics and preferences, allowing marketers to better target these customers and create personalized communication strategies.
- Outlier model: This model works by flagging out of the usual behavior. For example, banks may use this data to identify frauds. Or an e-commerce company may use this model to identify unusual spikes in revenue and identify potential fraud.
- Forecasting model: This model utilizes historical data, specifically those with a numerical value, to make future predictions. For example, a retailer may predict the footfall in the next week, and an e-commerce store may predict the number of orders or ideal price for a product based on what has worked in the past.
- Clustering model: Based on certain attributes or characteristics, customers can be clustered or
divided into segments for better targeting. This could be geolocation or a customer who churns based on certain criteria.
In essence, the advancement of AI is giving a new depth to predictive analytics, making it highly valuable for marketers. However, before venturing into any predictive analytics venture, it’s crucial to identify the need to build these models, how they will improve marketing and business decisions, and what data needs to be extracted. Doing so will ensure proper utilization of resources, creating an enriching customer experience, enhancing sales and marketing, and ensuring business continuity.